PLUM (Henry et al., 2022; Alexander et al., 2018) simulates the demand and trade of food goods (e.g. cereals, oil crops, pulses, starchy roots, sugar, fruits and vegetables, wood, and dairy and meat products from ruminant livestock and monogastric livestock) and forest products based on least-cost optimization principles by adjusting commodity prices instead of assuming market equilibrium, allowing short-term surplus and deficits. This includes the costs of irrigation, fertilizer use and management intensity (e.g. pesticide and machinery use). PLUM constrains irrigation use from water availability at the basin level derived from the LPJ-GUESS run-off outputs, after adjusting for other uses and environmental limitations. Therefore, changes in water resources as well as plant requirement under future climates can drive adaptation responses in land management. PLUM captures the relationship between food demand in each country and income and food prices using a Modified, Implicit, Directly Additive Demand System (MAIDADS) approach (Preckel et al., 2010; Gouel and Guimbard, 2018). Prices are endogenous in PLUM and are adjusted through international commodity trade imbalances, while populations and country incomes are exogenously prescription often using SSPs scenarios (O’Neill et al., 2014).
Further information available in selected papers listed below.
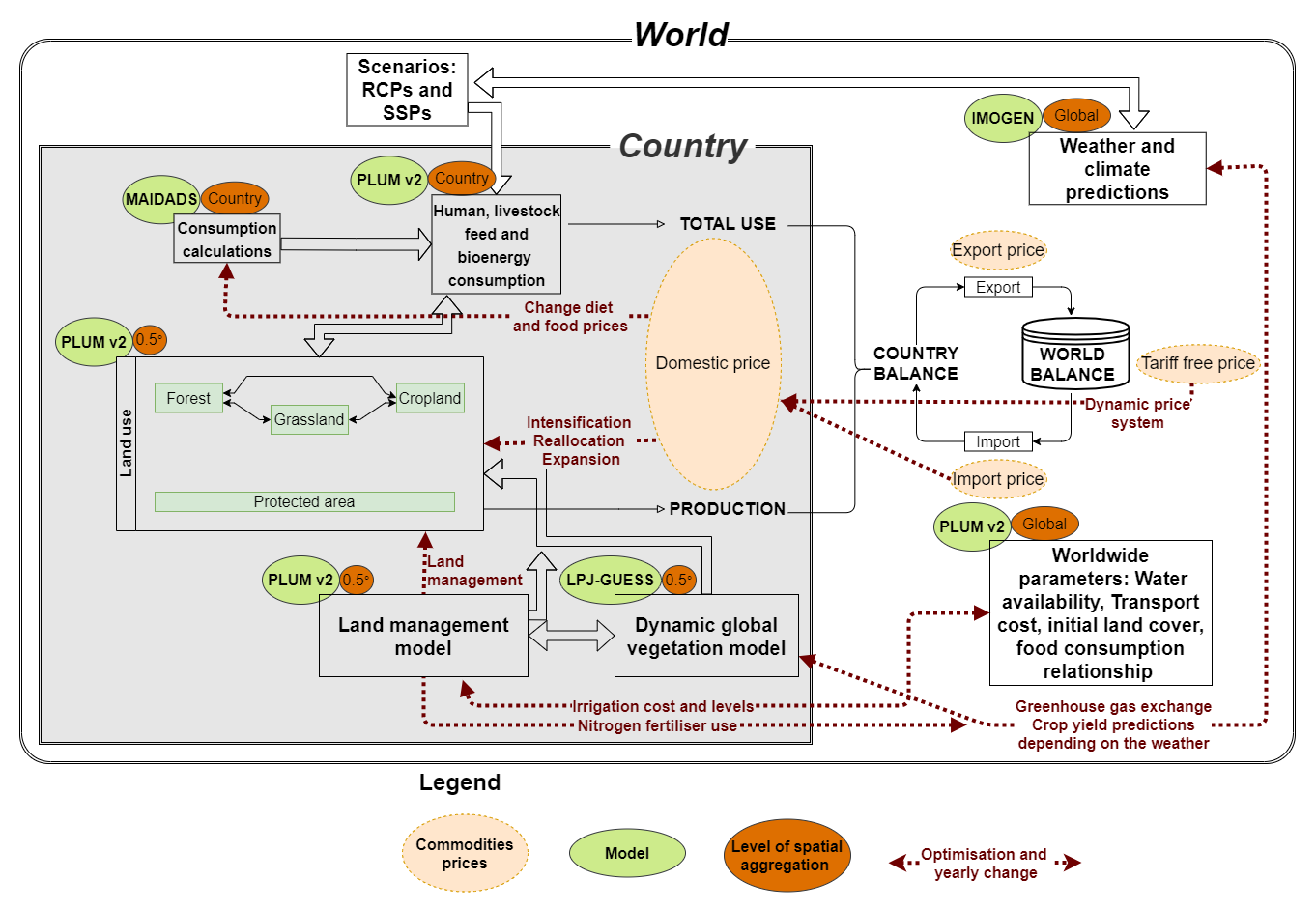
Selected references
Alexander P, Arneth A, Henry R, Maire J, Rabin S, Rounsevell M (2023) High energy and fertilizer prices are more damaging than food export curtailment from Ukraine and Russia for food prices, health and the environment.
Henry R, Arneth A, Jung M, Rabin R, Rounsevell M, Warren F & Alexander P (2022) Global and regional health and food security under strict conservation scenarios. Nature Sustainability.
Alexander, P., Rabin, S., Anthoni, P., Henry, R., Pugh, T.A.M., Rounsevell, M.D.A. & Arneth, A. (2018). Adaptation of global land use and management intensity to changes in climate and atmospheric carbon dioxide. Global Change Biology, 24(7), 2791-2809, doi.org/10.1111/gcb.14110
LPJ-GUESS (Smith et al., 2014) is a process-based, dynamic global vegetation model (DGVM) that simulates global and regional dynamics and composition of vegetation in response to changes in climate, land-use change, atmospheric CO2, and nitrogen. The model distinguishes between natural, pasture and cropland, and managed forest land-cover types, all of which include C–N dynamics (Olin et al., 2015a; Smith et al., 2014). Vegetation dynamics in natural land cover are characterized by the establishment, competition, and mortality of 12 plant functional types (PFTs) in a number of replicate patches. Physiological processes like photosynthesis, autotrophic, and soil heterotrophic respiration are calculated in natural and managed ecosystems; a set of carbon allocation rules determines plant growth. Plant establishment, growth, mortality, and decomposition, as well as their response to resource availability (light, nutrients, water), modulate seasonal and successional population dynamics arising from a carbon allocation trade-off. Vertical forest structure is accounted for by the use of different age classes for woody PFTs.
In managed ecosystems, pastures are populated by C3 or C4 grasses which are annually harvested (Lindeskog et al., 2013). Croplands are represented by prescribed fractions of five crop functional types (CFTs), which are moderately tilled, fertilized, and harvested (Olin et al., 2015a), and are prescribed to be either irrigated or rain-fed. The CFT yields are used as input to PLUM. Managed forests can be thinned, clear-felled or selectively harvested.
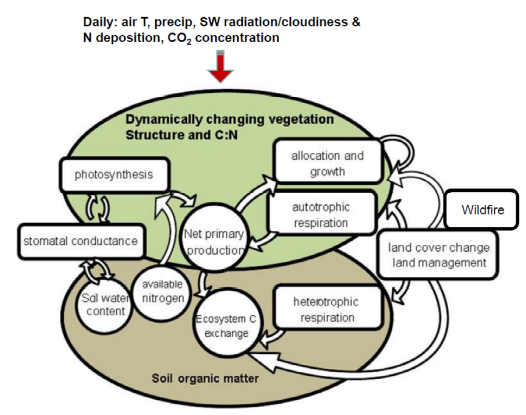
Selected references
Olin, S., et al., 2015. Modelling the response of yields and tissue C:N to changes in atmospheric CO2 and N management in the main wheat regions of western Europe. Biogeosciences 12, 2489-2515, 10.5194/bg-12-2489-2015.
Smith, B., et al., 2014. Implications of incorporating N cycling and N limitations on primary production in an individual-based dynamic vegetation model. Biogeosciences 11, 2027-2054, 10.5194/bg-11-2027-2014.
Madingley (Harfoot et al., 2014) is a process- and individual-based model of ecosystems. It aims to simulate the dynamics of all heterotrophic organisms in ecosystems. Madingley is different from existing ecological or biodiversity models as it includes both marine and terrestrial ecosystems, and marine and terrestrial human pressures – fisheries, agriculture, and climate change, for example. It is global in scope but can be applied regionally or nationally, and allows for unpredictable behaviour to emerge – meaning that food webs can shift and ecosystems can radically alter. The model is defined at the level of individual organisms, which are defined according to functional traits. All organisms undergo a set of fundamental ecological processes. Each organism undergoes metabolism to meet life costs, is predated upon by other organisms, eats itself from either plant or animal resources and if it can meet its metabolic costs, it will grow. When mature, organisms can reproduce offspring individuals into the model. Organisms are also subject to non-predation mortality in the form of starvation, senescence and background causes, such as disease. Lastly, organisms can move from their current location (a grid-cell) to another cell. As a result of these individual-level processes, higher-level properties of the ecosystem emerge from the model.
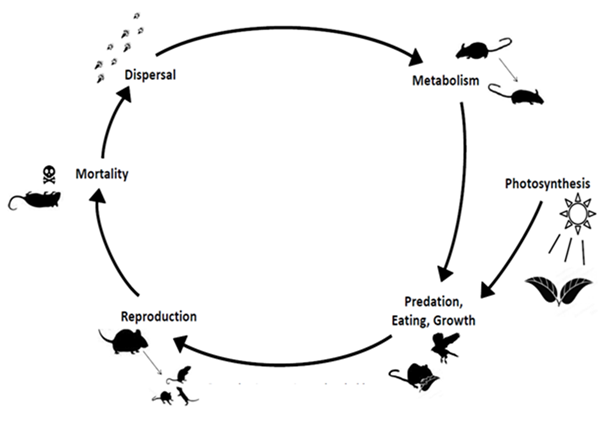
Selected references
Harfoot, M. B. J. et al. (2014), Emergent patterns of ecosystem structure and function from a mechanistic general ecosystem model. PLoS Biology, doi: 10.1371/journal.pbio.1001841
Bartlett, L., T. Newbold, D. Tittensor, D. Purves & M. Harfoot, (2016) Ecosystem responses to patterns of land use in a General Ecosystem Model, in review, Proc. Roy. Soc. B, doi: 10.1098/rspb.2016.1027.
IMOGEN (Huntingford et al., 2010) is an intermediate complexity climate system emulator to evaluate the impacts of climate change on the terrestrial surface. IMOGEN uses a pattern-scaling approach to climate change, which drives a gridded land surface and vegetation model MOSES/TRIFFID. The structure allows extrapolation of General Circulation Model (GCM) simulations to different future pathways of greenhouse gases, including rapid first-order assessments of how the land surface and associated biogeochemical cycles might change. Evaluation of how new terrestrial process understanding influences such predictions can also be made with relative ease.
Huntingford, C., Booth, B. B. B., Sitch, S., Gedney, N., Lowe, J. A., Liddicoat, S. K., Mercado, L. M., Best, M. J., Weedon, G. P., Fisher, R. A., Lomas, M. R., Good, P., Zelazowski, P., Everitt, A. C., Spessa, A. C., and Jones, C. D.: IMOGEN: an intermediate complexity model to evaluate terrestrial impacts of a changing climate, Geosci. Model Dev., 3, 679–687, https://doi.org/10.5194/gmd-3-679-2010, 2010.
CRAFTY (Brown et al., 2019) is a large-scale, agent-based modelling (ABM) framework for the simulation of land use change (CRAFTY: Competition for Resources between Agent Functional Types). It is designed to allow efficient but powerful simulation of a wide range of land uses across large geographical extents, based on the decision-making of simulated land managers who generate a variety of ecosystem services. It is fully open-source and can be used without the need for any programming. The basic framework is described in Murray-Rust et al. 2014.
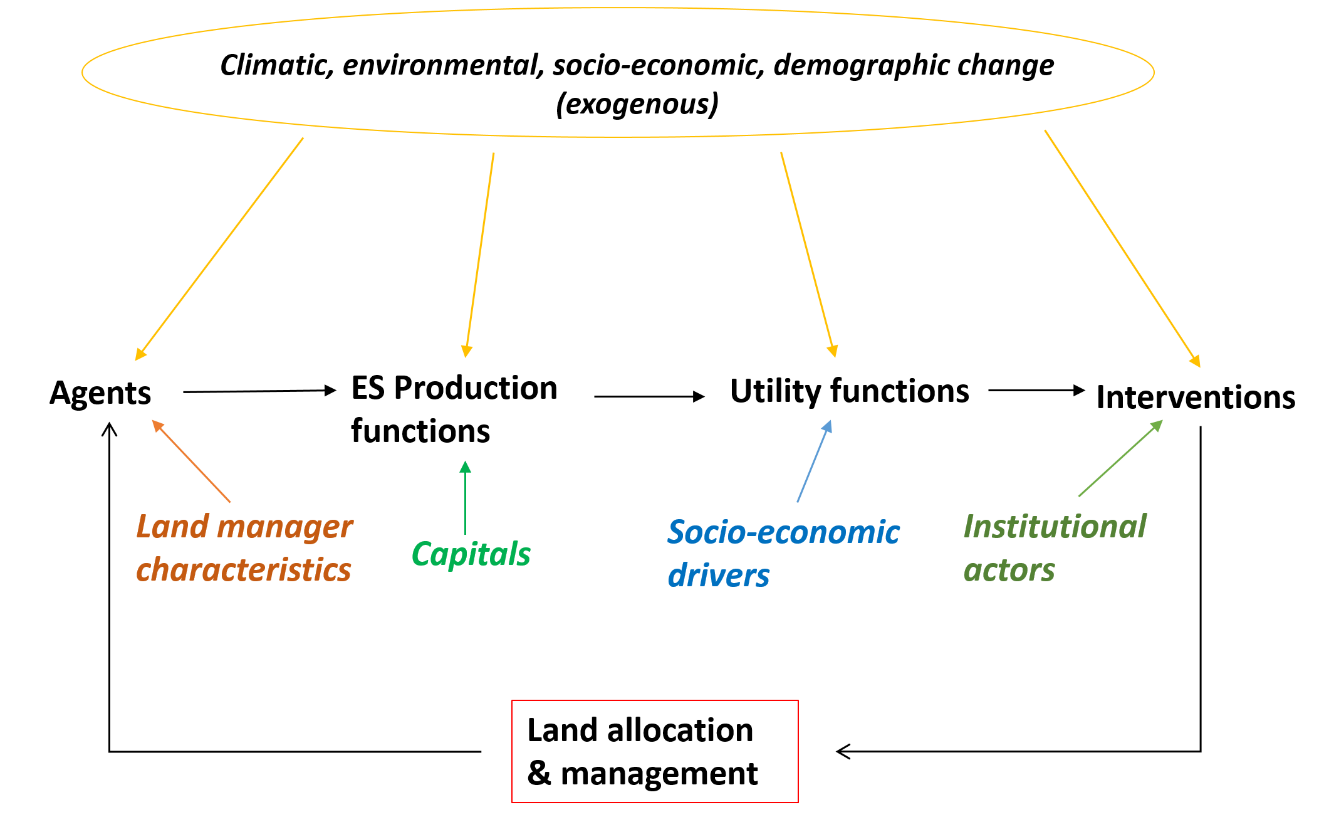
Selected references
Brown, C., Seo, B., & Rounsevell, M. (2019). Societal breakdown as an emergent property of large-scale behavioural models of land use change. Earth System Dynamics, 10, 809-845. https://doi.org/10.5194/esd-10-809-2019
Murray-Rust, D., Brown, C., van Vliet, J., Alam, S. J., Robinson, D. T., Verburg, P. H., & Rounsevell, M. (2014). Combining agent functional types, capitals and services to model land use dynamics. Environmental Modelling and Software, 59, 187–201. https://doi.org/10.1016/j.envsoft.2014.05.019